Application of machine learning algorithms to COSMIC-2 QC metrics
Maggie
Sleziak-Sallee
UCAR
Poster
The COSMIC Data Analysis and Archive Center (CDAAC) is an end-to-end processing and analysis system for ground- and space-based Global Navigation Satellite System (GNSS) data focusing on radio occultation (RO) applications. We process data and publish products from a variety of space missions, including FORMOSAT-7/COSMIC-2 (C2), in near real-time, post-processing, and re-processing modes. Near real-time products are delivered to operational centers for assimilation into weather and space weather analysis and prediction systems.
In this presentation we apply statistical inference and machine learning technology to a 3-month C2 neutral atmosphere retrieval dataset comprised of approximately 430K profiles. The dataset includes metrics such as geographic location, local time, signal-to-noise ratio, altitude range, bending angle noise from 60-80km, and others. We analyze correlations between all input parameters and correlation with our standard processing quality control (standard-QC) determination of whether a profile is “good” or “bad”. We then apply different machine learning classification algorithms to predict good and bad profiles from the set of input parameters. The set profiles determined as bad by standard-QC along with their ML classification results are then compared to numerical weather prediction analysis products. Our goal is to utilize ML to gain a deeper understanding of whether certain metrics can be used to optimize our standard bending angle retrieval quality control procedures.
In this presentation we apply statistical inference and machine learning technology to a 3-month C2 neutral atmosphere retrieval dataset comprised of approximately 430K profiles. The dataset includes metrics such as geographic location, local time, signal-to-noise ratio, altitude range, bending angle noise from 60-80km, and others. We analyze correlations between all input parameters and correlation with our standard processing quality control (standard-QC) determination of whether a profile is “good” or “bad”. We then apply different machine learning classification algorithms to predict good and bad profiles from the set of input parameters. The set profiles determined as bad by standard-QC along with their ML classification results are then compared to numerical weather prediction analysis products. Our goal is to utilize ML to gain a deeper understanding of whether certain metrics can be used to optimize our standard bending angle retrieval quality control procedures.
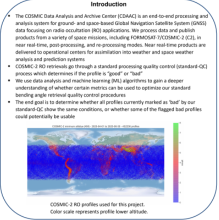
Poster PDF