Automated cloud top detection using machine learning algorithm on radio occultation profiles
Riccardo
Biondi
Università degli Studi di Padova, Dipartimento di Geoscienze, Padova, Italy
Poster
The bending angle anomaly, retrieved from radio occultation profiles, has been widely applied in previous research to determine the cloud top height of tropical cyclones and more recently the top height of volcanic clouds. This methodology has proven to be very accurate in altitude estimations, but it is time consuming. The objective of this work is to develop an algorithm able to acquire near real time RO profiles and to automatically determine the height of dense clouds in the upper troposphere and lower stratosphere.
In this work we collocated the GNSS radio occultations with volcanic clouds, tropical cyclones and clear sky data to build a statistically relevant training dataset for the machine learning algorithm. The target cloud top height of the algorithm is provided by an elaboration of the Cloud-Aerosol Lidar with Orthogonal Polarization (CALIOP) backscatter. The algorithm receives in input the CALIOP cloud top height and the bending angle anomaly of different atmospheric layers, learns the bending angle variations as a function of the altitude and how to identify the discontinuities due to the presence of a cloud, and provides in output an estimation of the cloud top height based on the bending angle profile.
The dataset includes more than one thousand collocations between RO and clouds in the period 2006-2015. From the entire dataset we have randomly selected 80% of the collocations used as training, 20% used as testing, and applied the cross-validation technique in order to validate the model performance to an independent dataset that was not used in estimating it. The output of the algorithm will contribute to the development of an already existing early warning system.
In this work we collocated the GNSS radio occultations with volcanic clouds, tropical cyclones and clear sky data to build a statistically relevant training dataset for the machine learning algorithm. The target cloud top height of the algorithm is provided by an elaboration of the Cloud-Aerosol Lidar with Orthogonal Polarization (CALIOP) backscatter. The algorithm receives in input the CALIOP cloud top height and the bending angle anomaly of different atmospheric layers, learns the bending angle variations as a function of the altitude and how to identify the discontinuities due to the presence of a cloud, and provides in output an estimation of the cloud top height based on the bending angle profile.
The dataset includes more than one thousand collocations between RO and clouds in the period 2006-2015. From the entire dataset we have randomly selected 80% of the collocations used as training, 20% used as testing, and applied the cross-validation technique in order to validate the model performance to an independent dataset that was not used in estimating it. The output of the algorithm will contribute to the development of an already existing early warning system.
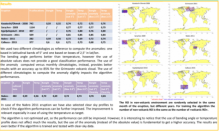
Poster PDF