Using Machine Learning to Detect Cloud Signatures in COSMIC- Radio Occultations
Stephen
Leroy
Atmospheric and Environmental Research
Poster
Radio occultation (RO) measurements have little direct sensitivity to clouds, but recent studies have shown that they may have an indirect sensitivity to thin, high clouds that are difficult to detect using conventional passive space-based cloud sensors. We apply two machine learning (ML) techniques to RO data to classify the presence or absence of clouds in 500-m vertical layers. The techniques are an XG-boosted random forest classification algorithms and a feed-forward neural network. Both algorithms are trained using COSMIC-2 RO profiles of bending angle and refractivity as the input data and cloud-truth as derived from the imagery of the Advanced Baseline Imager (ABI) on board GOES-R. ABI imagery in the visible and near-infrared, in conjunction with GFS forecasts of water vapor, provides a variety of cloud products at 10-minute cadence and horizontal and vertical resolutions of 500 m from geostationary orbit over the Americas. We use elements of signal detection theory – confusion matrices and receiver operator characteristic (ROC) curves – to evaluate the performance of the trained algorithms, simultaneously attempting to minimize false positives and false negatives. The resulting algorithms are validated by applying them to segments of the data that are withheld from the training.
We have conducted three tests: a climatological test in which longitude, latitude, and season are the only inputs; relative humidity and the geographic variables only; and a “kitchen sink” test involving a large number of RO retrieved variables and GFS forecasts. For both the XGboost test and the neural network, we find that most of the information is contained in the climatology, with an accuracy – the accuracy being the total probability of a correct prediction – of 80%. Providing relative humidity increases the accuracy minimally to 82%. The “kitchen sink” provides no additional information. Future work will include variables used in other proposed tests such as the vertical derivative of the logarithm of refractivity.
We have conducted three tests: a climatological test in which longitude, latitude, and season are the only inputs; relative humidity and the geographic variables only; and a “kitchen sink” test involving a large number of RO retrieved variables and GFS forecasts. For both the XGboost test and the neural network, we find that most of the information is contained in the climatology, with an accuracy – the accuracy being the total probability of a correct prediction – of 80%. Providing relative humidity increases the accuracy minimally to 82%. The “kitchen sink” provides no additional information. Future work will include variables used in other proposed tests such as the vertical derivative of the logarithm of refractivity.
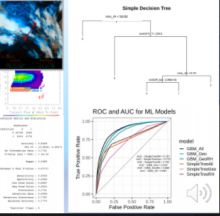
Poster PDF