Retrieving Temporally Dense GNSS-RO Profiles from Long Duration Balloon Platforms
Bryan
Chan
Night Crew Labs
Poster
Since 1995, space-based GNSS radio occultation (GNSS-RO) has made significant impacts on weather forecasting, climate prediction, and space weather research. However, it is difficult to remote sense the planetary boundary layer from space-based ROs due to geophysical and technological limitations (e.g., low signal-to-noise ratio, atmospheric ducting, and antenna gain loss). There is an opportunity to augment space-based GNSS-RO by using high-altitude, balloon-based platforms. These platforms represent a way to 1) provide persistent, on-demand RO data for specific regions of interest, and 2) reduce high costs and to expedite mission timeframes that are typically associated with LEO satellite constellations. GNSS-RO from a balloon platform allows adaptation to the ever-changing network of GNSS constellations, and the incorporation of the newest advances in GNSS receiver technology.
In this presentation, we discuss NCL’s GNSS-RO instrument and its implementation on a long-duration balloon mission. Data is collected for several weeks aloft in the stratosphere. A new balloon-borne bending angle retrieval algorithm that processes the GNSS-RO events is described. GNSS-RO results are assessed in quality, both in a case-by-case approach as well as in statistical analysis. The impact of balloon-based GNSS-RO on numerical weather prediction during balloon station-keeping (persistent hovering) events will be discussed. During these stationkeeping events, an unprecedented number of temporally dense regional RO soundings are collected. We also discuss the research to operations (R2O) developments needed to fully deploy an operational network of balloon-borne receivers. With these system changes, we illustrate how our GNSS-RO data may be assimilated into numerical weather predictions in the near future.
In this presentation, we discuss NCL’s GNSS-RO instrument and its implementation on a long-duration balloon mission. Data is collected for several weeks aloft in the stratosphere. A new balloon-borne bending angle retrieval algorithm that processes the GNSS-RO events is described. GNSS-RO results are assessed in quality, both in a case-by-case approach as well as in statistical analysis. The impact of balloon-based GNSS-RO on numerical weather prediction during balloon station-keeping (persistent hovering) events will be discussed. During these stationkeeping events, an unprecedented number of temporally dense regional RO soundings are collected. We also discuss the research to operations (R2O) developments needed to fully deploy an operational network of balloon-borne receivers. With these system changes, we illustrate how our GNSS-RO data may be assimilated into numerical weather predictions in the near future.
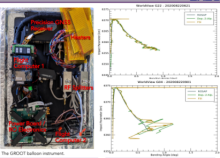
Poster PDF