Ocean Model Development, Data-driven Parameterizations, and Machine Learning in Ocean Models of the Earth System Workshop
A Joint CLIVAR Ocean Model Development Panel and COMMODORE Workshop
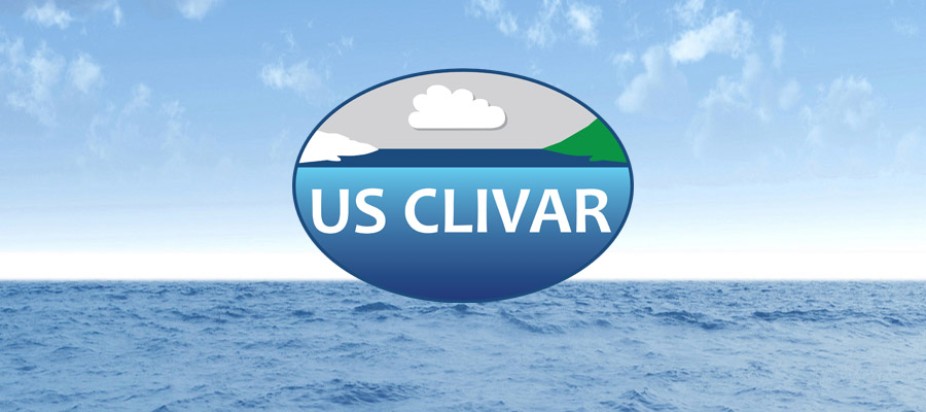
8:00 am – 5:00 pm MDT
Location
NSF NCAR Mesa Lab
Boulder, CO
Background
Dynamical core formulation, numerical methods, and spatial resolution have all made advances in recent years with ramifications for the global earth system models. Nevertheless, by virtue of the finite resolution imposed by limited computational resources, numerical models still require the parameterization of missing, unresolved, or poorly resolved processes. Without sub-grid parameterizations models develop large biases and/or become inaccurate for prediction. The workshop will therefore have a focus on both numerics and parameterizations, and in the context of both regional and global scale applications.
Parameterizations have traditionally been developed with a blend of empiricism and theory. Recently, machine learning as a phenomenon has been transforming science and the world at large. At the foundation of machine learning is the use of functions that model data and is fundamentally an empirical approach. The alignment of the data-driven aspects of machine learning with the needs of parameterizations has led to growing interest in using machine learning for parameterization development. Beyond the data-driven opportunities for parameterizations, the prominence and computational demands of machine learning represent a potentially disruptive moment in the history of ocean modeling and the course for the future of existing models is unclear. Making use of machine learning in ocean models seems to be an imminent transition so we aim to peer into the future to seek a path forward for the adoption of the new techniques in both parameterization, modeling more broadly, and analysis.