A Deep Generative+Self-Supervised Approach that Uses Physical Quantities to Generate and Retrieve Solar Images
Subhamoy
Chatterjee
Southwest Research institute, Boulder, CO, USA
Andrés Muñoz-Jaramillo, Southwest Research institute, Boulder, CO, USA
Anna Malanushenko, High Altitude Observatory, NCAR, Boulder, CO, USA
Anna Malanushenko, High Altitude Observatory, NCAR, Boulder, CO, USA
Poster
Deep generative models have shown immense potential in generating unseen data that has properties of real data. These models learn complex data-generating distributions starting from a smaller set of latent dimensions. However, generative models have encountered skepticism in scientific domains due to the disconnection between generative latent vectors and scientifically relevant quantities. In this study, we integrate three types of machine learning models (including a self-supervised model) to generate high-quality solar images in a physically interpretable manner and use those as a query to find matching patches in real observations. We find that the retrieved real data shares the same physical properties as the generated query. This elevates Generative AI from a means-to-produce artificial data to a novel tool for scientific data interrogation. This approach applies to any modality of astronomical data and will enable efficient exploration of PUNCH's coronal imagery from physical space.
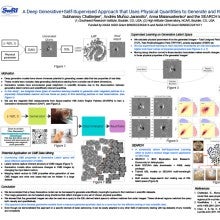
Poster PDF
Meeting homepage