Exploring Performance Trends of Simulated Real-time Solar Flare Predictions
Griffin
Goodwin
Georgia State University
Poster
In this study, our goal is to explore the factors that influence machine learning-based predictions of solar flares in a simulated operational environment. Using Georgia State University’s Space Weather Analytics for Solar Flares benchmark dataset (doi:10.7910/DVN/EBCFKM), we investigate the impact of training window type, solar cycle, and solar X-ray background flux on support vector machine, decision tree, and feed-forward neural network model performance.
We train classifiers using three window types: stationary, rolling, and expanding. The stationary window utilizes a single set of data available before the forecasting instance, which remains constant throughout the testing period. The rolling window utilizes data from a constant time interval before the forecasting instance, which moves with the testing period. Finally, the expanding window utilizes all available data before the forecasting instance. To our surprise, skill scores were comparable across all window/classifier combinations. We can conclude that training with more than 1.75 years’ worth of data does not significantly improve the quality of predictions.
Additionally, a moderate positive correlation exists between the X-ray background flux and the percent of non-flaring false positive predictions. Likely related, a moderate negative correlation exists between a model’s true skill statistic score and the number of sunspots present. This suggests that the X-ray background flux and solar cycle phase have a large influence on forecasting.
Lastly, to gain better intuition about our results, we present a novel visualization that provides insightful analysis into the temporal performance of a classifier.
We train classifiers using three window types: stationary, rolling, and expanding. The stationary window utilizes a single set of data available before the forecasting instance, which remains constant throughout the testing period. The rolling window utilizes data from a constant time interval before the forecasting instance, which moves with the testing period. Finally, the expanding window utilizes all available data before the forecasting instance. To our surprise, skill scores were comparable across all window/classifier combinations. We can conclude that training with more than 1.75 years’ worth of data does not significantly improve the quality of predictions.
Additionally, a moderate positive correlation exists between the X-ray background flux and the percent of non-flaring false positive predictions. Likely related, a moderate negative correlation exists between a model’s true skill statistic score and the number of sunspots present. This suggests that the X-ray background flux and solar cycle phase have a large influence on forecasting.
Lastly, to gain better intuition about our results, we present a novel visualization that provides insightful analysis into the temporal performance of a classifier.
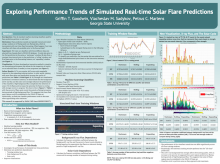
Poster PDF
Poster category
Solar and Interplanetary Research and Applications
Meeting homepage