A Federated Distributed Learning Benchmark for solar wind speed forecasting using solar EUV images
Filip
Svoboda
University of Cambridge
Poster
Distributed training is the future of on-board computation in space as it offers scalability, resilience, and flexibility that can not be matched by a centralized setup. In the communication space it trades-in the cost of a full-dataset aggregation for that of an intermittent exchange of training messages. This work first explores the resource cost landscape of centralized training and a number of distributed variants. Federated learning, we observe, greatly lowers the communication cost of message passing relative to its distributed peers. It is, therefore, chosen for closer examination in the second part of this work. When used on the state of the art transformer model for solar wind speed prediction (Svoboda, Brown et al., 2022) and the Extreme UV images taken by the Solar Dynamics Observatory (OmniWeb, 2023) it retains the performance of the centralized model under both IID and non-IID conditions, while offering significant communication savings. Our extensive battery of experiments shows that the observed results are robust to a wide array of changes in the client count and the degree of data distribution heterogeneity. Furthermore, our results give materially significant recommendations relevant to the design of future missions as they identify a substantial trade-off between the benefits of adding new data, and the cost of adding more clients.
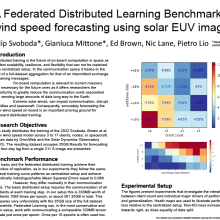
Poster PDF
Poster category
Solar and Interplanetary Research and Applications
Meeting homepage