Imbalanced regression and large event prediction: application on whistler-mode chorus using a neural network
Xiangning
Chu
Laboratory for Atmospheric and Space Physics, University of Colorado Boulder, Boulder, Colorado, USA
Poster
Real-world data sets often exhibit imbalanced distributions, which have significantly more data or observations in a specific range of values than the other ranges. For example, space physics data sets, such as geomagnetic indices, relativistic electron fluxes in Earth's radiation belt, and the occurrence and amplitude of solar flares, are typically imbalanced. This is the too-often-too-quiet challenge, one of the fundamental problems in space physics and space weather, and is also a general problem in machine learning. For example, the electron density and plasma fluxes in the Earth's radiation belts can be accurately modeled in our previous studies [Bortnik et al., 2016, 2018; Chu et al., 2017a,b; 2021; Ma et al., 2022a, b]. However, the ML-based models of the plasma waves are usually biased due to the too-often-too-quiet problem both in numerical simulations and observations [Ma et al., 2018; Camporeale et al., 2019; Guo et al., 2021].
We developed a method to solve this problem and applied it to the whistler-mode chorus waves in the Earth's radiation belt. The ML-based wave model used a neural network approach, which takes geomagnetic indices as input and prediction the wave power. As a result, the model can predict not only the quiet time values but also large events. The fact demonstrates that the model provides reliable and stable predictions when the too-often-too-quiet problem is solved. This method of imbalanced regression has wide applications in space physics/weather and a wider field of machine learning techniques.
We developed a method to solve this problem and applied it to the whistler-mode chorus waves in the Earth's radiation belt. The ML-based wave model used a neural network approach, which takes geomagnetic indices as input and prediction the wave power. As a result, the model can predict not only the quiet time values but also large events. The fact demonstrates that the model provides reliable and stable predictions when the too-often-too-quiet problem is solved. This method of imbalanced regression has wide applications in space physics/weather and a wider field of machine learning techniques.
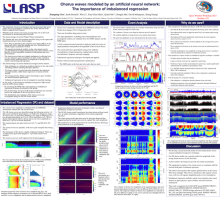
Poster PDF
Poster category
Geospace/Magnetosphere Research and Applications
Meeting homepage