Improving Space Weather Predictions with Data-driven Models of the Solar Atmosphere and Inner Heliosphere
Nikolai
Pogorelov
Univsersity of Alabama in Huntsville
Poster
To address Objective II of the National Space Weather Strategy and Action Plan 'Develop and Disseminate Accurate and Timely Space Weather Characterization and Forecasts' and US Congress PROSWIFT Act 116–181, our team has been developing a new suite of open-source software that would ensure substantial improvements of Space Weather (SWx) predictions. On the one hand, the focus is on the development of data-driven models. On the other hand, each individual component of our software is aimed to have higher accuracy with a subtantially improved performance. This is done by the application of new computational technologies and enhanced data sources. The development of such software paves way for improved SWx predictions accompanied with an appropriate uncertainty quantification. This will make it possible to forecast hazardous SWx effects on the space-borne and ground-based technological systems, and on human health. Our models involve (1) a new, open-source solar magnetic flux model (OFT), which evolves information to the back side of the Sun and its poles, and updates the model flux with new observations using data assimilation methods; (2) a new potential field solver (POT3D) associated with the Wang-Sheeley-Arge coronal model, and (3) a new adaptive, 4-th order of accuracy solver (HelioCubed) for the Reynolds-averaged MHD equations implemented on mapped multiblock grids (cubed spheres). We describe the software and results obtained with it, including the application of machine learning to modeling coronal mass ejections, which makes it possible to improve SWx predictions by decreasing the time-of-arrival mismatch. The tests show that our software is formally more accurate and performs much faster than its predecessors used for SWx predictions.
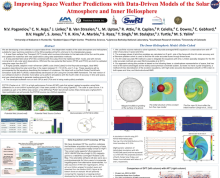
Poster PDF
Poster category
Solar and Interplanetary Research and Applications
Meeting homepage