A Machine Learning Ecosystem for Filament Analysis - Phase I: A Manually Annotated Dataset of Filaments
Samuel
McDonald
Department of Computer Science, Georgia State University
Poster
Detecting and classifying solar filaments is critical in forecasting Earth-affecting transient solar events, including large solar flares and coronal mass ejections. Undetected, these space weather events can cause catastrophic geomagnetic storms resulting in substantial economic damage and death. A network of ground-based observatories, named the Global Oscillation Network Group, was created to provide continuous observation of solar activity. However, parsing the large volume of image data continuously streaming in from GONG tests the limitations of human analysis and presents challenges for space weather research. To address this, we proposed the Machine Learning Ecosystem for Filament Detection (MLEcoFi), an NSF-funded, multiyear project that will produce an open-source collection of filament data and computer vision software for space weather research. In cooperation with NSO, MLEcoFi will assist in automatically detecting, classifying, localizing, and segmenting solar filaments in full-disk H-alpha images. The present phase of research aims to produce a dataset of thousands of GONG H-alpha images, with all filaments’ chirality, bounding box, and segmentation mask manually annotated following strong quality assurance standards, advancing research of filaments and filament-related topics. This dataset, as the first MLEcoFi product, will aid in ongoing and future development of products, including a chirality-aware filament data augmentation engine, high-precision image segmentation loss function, deep neural network segmentation and classification model, and filament detection module that is planned for deployment into NSO’s live infrastructure for the research community. The MLEcoFi team is eager to present its progress and future milestones to obtain valuable feedback from the future users of this ecosystem.
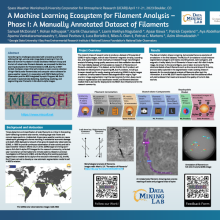
Poster PDF
Poster category
Solar and Interplanetary Research and Applications
Meeting homepage