MEMPSEP: A Multivariate Ensemble of Models for Probabilistic forecast of SEP Occurrence and Properties
Maher
Dayeh
SwRI, San Antonio, Texas, USA/UTSA, Texas, USA
Poster
Solar flares, Coronal Mass Ejections (CMEs), and Solar Energetic Particles (SEPs) are among the key drivers of space weather in the near-Earth environment and beyond. While some CMEs and flares are associated with intense SEPs, some show no or little SEP association. Furthermore, there is no clear and consistent connection between the properties of SEPs observed at 1 au and their progenitors at or near the Sun. The latter is due to the complex environment that dominates SEP origin, acceleration, and transport in the interplanetary space. To date, robust long-term (hours to days) forecasting of SEP properties does not effectively exist and the search for such development continues.
In this work, we present an ensemble of neural networks that uses both remote and in-situ data from multiple sources to predict the true probability of SEP occurrence and its properties such as peak intensity within different energy pass-bands, event onset time, and duration. We show that incorporating the SEP occurrence probability as a weighting factor into the regression can tighten the prediction of event properties on the test set. We also show how the uncertainty estimated through the ensemble of models enables robust forecasting decisions for unseen data. This work is fully supported by the O2R program (Grant no. 80NSSC20K0290).
In this work, we present an ensemble of neural networks that uses both remote and in-situ data from multiple sources to predict the true probability of SEP occurrence and its properties such as peak intensity within different energy pass-bands, event onset time, and duration. We show that incorporating the SEP occurrence probability as a weighting factor into the regression can tighten the prediction of event properties on the test set. We also show how the uncertainty estimated through the ensemble of models enables robust forecasting decisions for unseen data. This work is fully supported by the O2R program (Grant no. 80NSSC20K0290).
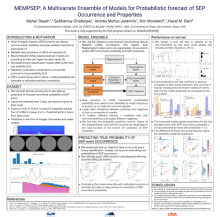
Poster PDF
Poster category
Solar and Interplanetary Research and Applications
Meeting homepage