Predicting Solar Proton Events with Random Hivemind Models: An Application of Ensemble Deep Learning
Patrick
O'Keefe
New Jersey Institute of Technology
Poster
Deep learning has become a popular method of predicting solar proton events (SPEs) due to its malleability and its adaptability to new data. However, a series of parameters passed to a conventional neural network (CoNN) may be rather arbitrary, especially if there is no surefire way to decide how to program hyperparameters for a given dataset and the chaotic nature of neural networks. The random hivemind (RH) addresses this concern by having multiple neural network estimators with random permutations of features take part in decisions. Learning rates and the maximum numbers of epochs may be boosted or attenuated depending on how important all features used by a given estimator are, but all other hyperparameters remain the same across estimators. This allows one to quickly see whether consistent class decisions can be made by multiple neural networks with the same sets of hyperparameters, with random subsets of features chosen to force variation in how data are predicted by each, placing the quality of the data and hyperparameters into focus. The effectiveness of RH in the predictions of SPEs is demonstrated by comparing it to that of using both CoNN and the traditional committee-based approach used in ensemble deep learning through experimentation. Our results demonstrate that RH is capable of meeting or outperforming the CoNN and the identical neural network committees, and demonstrates promising results with respect to the "all-clear'' prediction of SPEs.
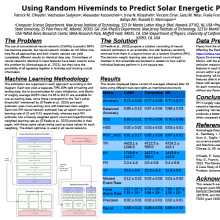
Poster PDF
Poster category
Solar and Interplanetary Research and Applications
Meeting homepage