Solar flare nowcasting using multi-wavelength SDO/AIA data
Paolo
Massa
Department of Physics & Astronomy, Western Kentucky University (KY, USA)
Poster
We consider the development of machine learning models to forecast the occurrence of solar flares a few tens of minutes in advance. Such results can be used to support projects such as the NASA Solar Flare Sounding Rocket Campaign in Spring 2024, during which three rocket-borne instruments will be launched near-simultaneously to observe the evolution of a flare. Accurate advance prediction of the flare will enhance the chances of observing the flare in its impulsive/rise phase. We have trained Convolutional Neural Networks (CNNs) on images of active regions recorded by the Atmospheric Imaging Assembly on board the Solar Dynamics Observatory (SDO/AIA) in seven EUV wavelengths at several consecutive timesteps, forming four-dimensional (x,y,λ,t) “datacubes”. These datacubes contain information on both morphological and thermodynamic changes within the active regions, both of which are likely precursors of flaring activity, and hence have an enhanced predictive capability compared to the magnetograms that are usually employed. We show preliminary results obtained by training CNNs on a dataset of active region datacubes recorded between 2010 and 2018. We also discuss Fourier-based data compression techniques that can be used to significantly reduce the size of the dataset while retaining its essential morphological and thermodynamic information. We also describe the training of CNNs on Differential Emission Measure (DEM) datacubes that are constructed from the original (x,y,λ,t) datacubes by means of a novel Regularized Maximum Likelihood inversion algorithm.
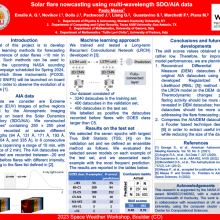
Poster PDF
Poster category
Solar and Interplanetary Research and Applications
Meeting homepage