Exploring the Re-Creation of the McIntosh Archive with Deep Learning
Ryan
Nguyen
Orion
Poster
The McIntosh Archive stands as a remarkable collection of hand-drawn solar Carrington maps meticulously crafted by Patrick McIntosh spanning from 1964 to 2009. McIntosh ingeniously amalgamated data from H-alpha, He-II 10830 Å, and photospheric magnetic measurements obtained from ground-based observatories and NASA satellites to create maps of the evolution of solar features during four solar cycles. In response to the gap left by the passing of Patrick McIntosh and the discontinuation of his solar Carrington maps, we have employed Machine Learning methods to replicate his map generation process. We discuss the utilization of Supervised Learning through a variational autoencoder (VAE), Unsupervised Learning via K-means clustering, and a novel Reinforcement Learning (RL) approach. Excitingly, the Supervised Learning model developed for Coronal Hole identification exceeded our performance expectations when validated against hand-drawn maps created by trained cartographers. It appears to yield results that are as good or better than previous machine learning approaches.
We have also used the same Supervised Learning approach to identify the PILs, filaments, and sunspots with the remaining two out of three solar image types, and obtained similar high quality results. The Supervised Learning approach is important because it could increase the speed at which Carrington Rotation maps can be generated, yet with minimal sacrifice in performance. This may mean they could be generated in real-time for use in Space Weather operations.
Results from a Reinforcement Learning approach will also be discussed, together with a concept for the fusion of both methods (Supervised Learning and Reinforcement Learning).
We have also used the same Supervised Learning approach to identify the PILs, filaments, and sunspots with the remaining two out of three solar image types, and obtained similar high quality results. The Supervised Learning approach is important because it could increase the speed at which Carrington Rotation maps can be generated, yet with minimal sacrifice in performance. This may mean they could be generated in real-time for use in Space Weather operations.
Results from a Reinforcement Learning approach will also be discussed, together with a concept for the fusion of both methods (Supervised Learning and Reinforcement Learning).
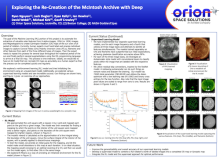
Poster PDF
Poster category
Solar and Interplanetary Research and Applications
Poster session day
Poster location
20
Meeting homepage