Improving Space Weather Forecasts Improving Space Weather Predictions with Data-Driven Models of the Solar Atmosphere and Inner Heliosphere
Nikolai
Pogorelov
The University of Alabama in Huntsville, Department of Space Science
Poster
We describe a newly-developed software suitable for near real-time predictions of the SW properties at Earth’s orbit and in the interplanetary space. It involves the following components.
1. A new Open surface Flux Transport (OFT) model which evolves information to the back side of the Sun and its poles and update the model flux with new observations using data assimilation methods.
2. A new potential field solver (POT3D) combined with the output from the traditional WSA model, and with remote coronal and in situ solar wind observations. WSA and the new potential field solver (PFSS and PFCS) are both be validated using the maps from the OFT. Due to its efficiency and versatility, it is especially efficient for ensemble modeling.
3. A highly parallel, adaptive mesh refinement (AMR) code (HelioCubed) for the Reynolds-averaged, ideal MHD system of equations between 0.1 au and 1–3 au. This system also involves equations describing the transport of turbulence. HelioCubed is built on Chombo 4 framework and and makes it possible to perform simulations with the fourth order of accuracy in time and space, and use cubed spheres to generate meshes around the Sun.
4. The developed software runs on both GPUs and CPUs and is being made publicly available.
5. We also apply machine learning techniques and demonstrate that their application is critical for accurate predictions of coronal mass ejection arrival at Earth.
1. A new Open surface Flux Transport (OFT) model which evolves information to the back side of the Sun and its poles and update the model flux with new observations using data assimilation methods.
2. A new potential field solver (POT3D) combined with the output from the traditional WSA model, and with remote coronal and in situ solar wind observations. WSA and the new potential field solver (PFSS and PFCS) are both be validated using the maps from the OFT. Due to its efficiency and versatility, it is especially efficient for ensemble modeling.
3. A highly parallel, adaptive mesh refinement (AMR) code (HelioCubed) for the Reynolds-averaged, ideal MHD system of equations between 0.1 au and 1–3 au. This system also involves equations describing the transport of turbulence. HelioCubed is built on Chombo 4 framework and and makes it possible to perform simulations with the fourth order of accuracy in time and space, and use cubed spheres to generate meshes around the Sun.
4. The developed software runs on both GPUs and CPUs and is being made publicly available.
5. We also apply machine learning techniques and demonstrate that their application is critical for accurate predictions of coronal mass ejection arrival at Earth.
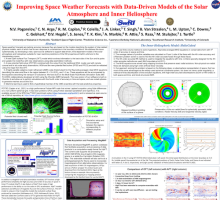
Poster PDF
Poster category
Solar and Interplanetary Research and Applications
Poster session day
Poster location
3
Meeting homepage