Operational Solar Flare Predictions and Evaluations using Deep Flare Net
Naoto
Nishizuka
National Institute of Information and Communications Technology
Poster
We have developed an operational solar flare prediction model using deep neural networks, named Deep Flare Net (DeFN), which is used in daily space weather forecast meetings in NICT (Nishizuka et al. 2018 ApJ, 2021 EPS). The DeFN model can predict occurrence probabilities and the largest level of flares occurring within the next 24 hr. We used magnetograms and EUV images taken by HMI and AIA onboard SDO during 2010-2015. DeFN can automatically detect active regions from magnetograms, extract 79 physical features from each region, and input them into deep neural networks to predict flares occurring in the next 24 hours. We evaluated the prediction results by TSS, and we found that DeFN succeeded in predicting flares with TSS=0.80 for >=M-class flares and 0.63 for >=C-class flares, which is better than human forecasting. Furthermore, we have operated DeFN since 2019, and it has been used for daily forecasting. We evaluated the operational prediction results in the period of 2019/1-2020/7, and we found that DeFN achieved TSS=0.82 for >=C-class flare predictions. Recently, we have evaluated the prediction results again in the period of 2019/1-2023/6, and we found that DeFN achieved TSS=0.70 (0.72) for >=M(C)-class flare predictions. In this presentation, we would like to introduce our DeFN model and operational results for five years.
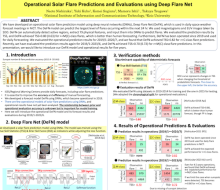
Poster PDF
Poster category
Solar and Interplanetary Research and Applications
Poster session day
Poster location
37
Meeting homepage