Pushing the Frontiers of Operational Geoelectric Hazard Modeling
Gabriel
Moraga¹
University of Colorado at Boulder
Poster
Space weather, and its effects on Earth, is a trending topic. Geoelectric fields are influenced by geomagnetic field variation caused by Earth's surrounding space weather environment. Geoelectric hazards from space weather can critically affect infrastructure, and the aftermath is economically costly. It is essential to enhance geoelectric hazard modeling by implementing a combination of research and machine learning. This will determine where and when geomagnetically induced currents (GICs) may impact critical infrastructure.
Organizations such as NOAA, USGS, NASA, NSF and international partners (NRCan) have contributed to an operational geoelectric field map data product. Currently, ground based stations across North America measure real-time surface geomagnetic field data, which is driven by natural-time ionosphere conductivities within the thermosphere. The current geoelectric field maps use the Spherical Elementary Current System (SECS) interpolation method, based on physical constraints of the magnetic field. Currently there is no machine learning method in place to fill missing data and flag outliers in real-time.
Our team created an algorithm in Python, using real-time data, which interpolates missing values and detects anomalies outside a standard deviation of 3 sigma. With the Seasonal Auto-Regressive Integrated Moving Average (SARIMA) anomaly detection model as the base, the newly created model will fill data gaps and flag anomalies. We generate more accurate geoelectric field maps using machine-learning algorithms that identify and replace missing data within the real-time data streams. The final machine learning model will be run in the CU’s Space Weather Technology Research and Education Center’s (TREC) cloud-based Testbed environment.
Organizations such as NOAA, USGS, NASA, NSF and international partners (NRCan) have contributed to an operational geoelectric field map data product. Currently, ground based stations across North America measure real-time surface geomagnetic field data, which is driven by natural-time ionosphere conductivities within the thermosphere. The current geoelectric field maps use the Spherical Elementary Current System (SECS) interpolation method, based on physical constraints of the magnetic field. Currently there is no machine learning method in place to fill missing data and flag outliers in real-time.
Our team created an algorithm in Python, using real-time data, which interpolates missing values and detects anomalies outside a standard deviation of 3 sigma. With the Seasonal Auto-Regressive Integrated Moving Average (SARIMA) anomaly detection model as the base, the newly created model will fill data gaps and flag anomalies. We generate more accurate geoelectric field maps using machine-learning algorithms that identify and replace missing data within the real-time data streams. The final machine learning model will be run in the CU’s Space Weather Technology Research and Education Center’s (TREC) cloud-based Testbed environment.
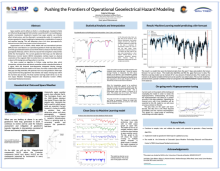
Poster PDF
Poster category
Geospace/Magnetosphere Research and Applications
Meeting homepage