Predicting Solar Energetic Particle Event Occurrences Using Explainable AI
Yuta
Kato
Fujitsu Limited
Poster
Solar Energetic Particle (SEP) Events, which occur in conjunction with solar flares (SFs) and coronal mass ejections (CMEs), have effects on both humans and space systems.
We conduct a classification task using Wide Learning, an explainable AI developed by Fujitsu, to explore the conditions of SFs with/without SEP Events. Wide Learning which is based on emerging pattern mining algorithms was originally developed in the field of Discovery Science, enables us to conduct classification tasks with exhaustive conditions.
We created 57 features from GOES and SDO satellites data and the physics-based three-dimensional extrapolated magnetic fields developed by Kusano et al. (2020). We classified SFs that meet > 10 MeV, > 10 pfu in the NOAA SWPC database during Solar Cycle 24 as positive samples, and all other SFs as negative samples. Due to the class imbalance, we fixed the positive flares and under sampled the negative flares to achieve a 1:3 ratio for each Flare class.
We conducted 100 trials with random replacements and our model demonstrates a True Skill Statistic (TSS) approximately about 0.4. For positive flares, we identified multiple useful conditions with numerical ranges which consist of the longitude, duration time, and history of SFs. These results suggest the potential for predicting SEP Event occurrences with real-time step-by-step alerts, and the ability to reference past cases that align with identified conditions. We also discuss the potential for SEP event forecasting using these conditions and future prospects.
We conduct a classification task using Wide Learning, an explainable AI developed by Fujitsu, to explore the conditions of SFs with/without SEP Events. Wide Learning which is based on emerging pattern mining algorithms was originally developed in the field of Discovery Science, enables us to conduct classification tasks with exhaustive conditions.
We created 57 features from GOES and SDO satellites data and the physics-based three-dimensional extrapolated magnetic fields developed by Kusano et al. (2020). We classified SFs that meet > 10 MeV, > 10 pfu in the NOAA SWPC database during Solar Cycle 24 as positive samples, and all other SFs as negative samples. Due to the class imbalance, we fixed the positive flares and under sampled the negative flares to achieve a 1:3 ratio for each Flare class.
We conducted 100 trials with random replacements and our model demonstrates a True Skill Statistic (TSS) approximately about 0.4. For positive flares, we identified multiple useful conditions with numerical ranges which consist of the longitude, duration time, and history of SFs. These results suggest the potential for predicting SEP Event occurrences with real-time step-by-step alerts, and the ability to reference past cases that align with identified conditions. We also discuss the potential for SEP event forecasting using these conditions and future prospects.
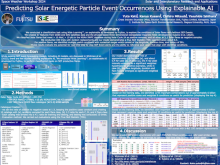
Poster PDF
Poster category
Solar and Interplanetary Research and Applications
Poster session day
Poster location
14
Meeting homepage