Understanding Plasma Flows in Solar Active Regions: a neural network based method for the recovery and analysis of coherent plasma structures in magnetically dominated environments
Matthew
Lennard
Department of Automatic Controls and Systems Engineering, The University of Sheffield, UK
Poster
High-energy solar events such as solar flares (SFs) and coronal mass ejections (CMEs) are the result of the build up of strong magnetic fields in active regions (ARs). Identifying plasma flows in ARs and understanding their structure and evolution are still challenging. Recently, a number of realistic simulations of sunspots have appeared, in particular, the R2D2 code has been used to simulate the evolution of a flux tube in its entirety in a box covering the whole convective region (see Hotta and Iijima 2020). What remains is the assimilation of data in models to support our understanding of observed transient solar phenomena.
In this work we train the neural network (NN) DeepVel (DV, see Asensio Ramos 2017) on R2D2 simulation data to reconstruct subgranular transverse flows in photospheric ARs. We then present a method for analysing these detailed DV estimated flows by seeking coherent structures in the flow by identifying flow barriers defined to be the most repelling and attracting structures in the flow, described by the finite-time Lyapunov exponent (FTLE, see e.g., Haller 2014).
We have found that DV is able to estimate transverse flows on length scales <1Mm. Flow structures surrounding pores have been found to be consistent with Evershed flows that have been identified in observations and that coherent structures, defined by FTLE ridges may be used to identify flows that are indicative of the presence of an AR emerging.
In this work we train the neural network (NN) DeepVel (DV, see Asensio Ramos 2017) on R2D2 simulation data to reconstruct subgranular transverse flows in photospheric ARs. We then present a method for analysing these detailed DV estimated flows by seeking coherent structures in the flow by identifying flow barriers defined to be the most repelling and attracting structures in the flow, described by the finite-time Lyapunov exponent (FTLE, see e.g., Haller 2014).
We have found that DV is able to estimate transverse flows on length scales <1Mm. Flow structures surrounding pores have been found to be consistent with Evershed flows that have been identified in observations and that coherent structures, defined by FTLE ridges may be used to identify flows that are indicative of the presence of an AR emerging.
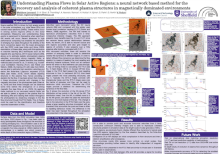
Poster PDF
Poster category
Solar and Interplanetary Research and Applications
Poster session day
Poster location
24
Meeting homepage